Charlotte Bax is the founder and CEO of Captur, a London based start-up that provides solutions for capturing and analysing images. By leveraging computer vision technology, Captur automates decision making based on the content of the image. For example, automating parking checks for micro-mobility companies such as Tier globally. Unfortunately, whenever there is a portal for people to upload content, there is also a need for moderation. Unitary interviewed Charlotte to explore how both computer vision and online safety challenges can show up in unexpected places.
How did you come up with the idea of Captur? What problem were you originally trying to address?
About six years ago, while collaborating on a project with Nasa, I learned an interesting fact. It’s now often faster for Google to use computer vision algorithms to predict what street views will look like versus getting the real image from a satellite feed. That’s pretty astounding, given the satellite image would take less than 3 seconds. And it got me thinking, how else can we use images to capture and understand the world?
At the same time, I was really interested in the on-demand economy and how our generation is increasingly moving from ownership to usership. The problem is, when you have something being moved around and shared, damage inevitably occurs. So how can businesses accurately capture the condition of shared cars, bikes, clothing, furniture, etc. remotely? Our first market is micro-mobility for two reasons. One, companies rely on manual reviews of images to confirm where users parked and can’t keep up with the huge volumes. Two, since the demand for e-bikes and scooters has exploded, these companies face new regulations from cities to ensure safe parking.
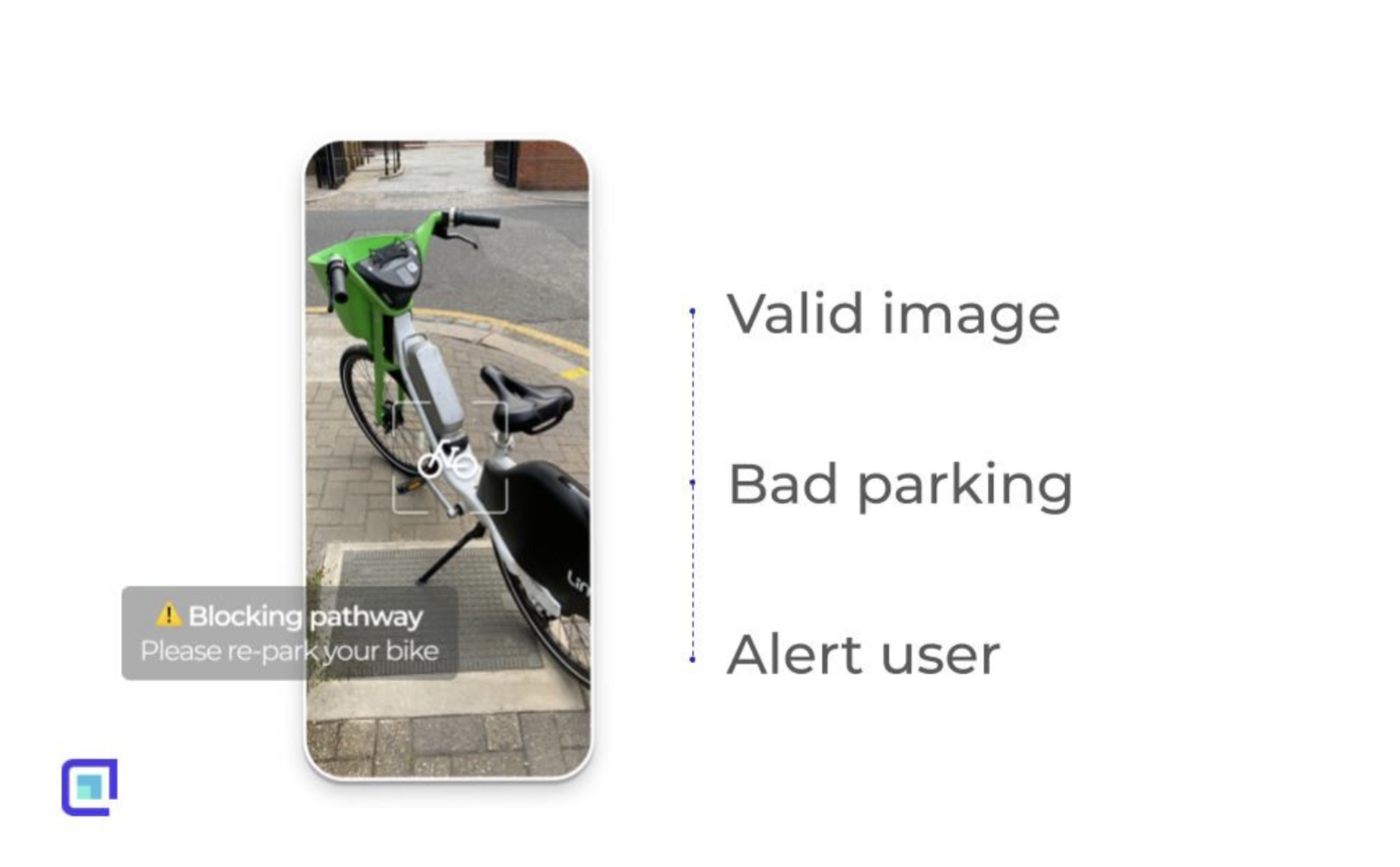
Why did you decide to apply computer vision specifically to the realm of road safety?
With computer vision, what we can do is understand not only the contents but also the context of information in an image. Not just detecting where a vehicle is, but how it is parked in relation to the environment. Then provide real-time feedback to improve the customer experience. We can also improve the current process by providing a more scalable and less subjective solution that works in any city globally.
So at Captur you are dealing with content that is created and uploaded by people. What challenges do you think are unique to this type of user generated content? And what does it mean to build visual AI in this space?
I think the big challenge for anyone in this space is the quality of data you use to train algorithms. And unfortunately we’ve seen that in real-world scenarios, people often take bad photos. And when images aren’t taken from the right angle, or are too close up or too blurry, the algorithms can’t confidently make a decision. So, there are lots of techniques that we’re working on to prevent inaccuracies and give real time guidance to users so they don’t submit something that’s invalid.
What do you think is the advantage of using AI technologies, like computer vision in daily operations, be it road safety, content moderation or any other industry?
I think it’s all about being able to scale a business process in a way that is both cost efficient and standardised. The larger the company gets, the more people are involved in a process, meaning more subjectivity and more unique ways to do things. So it ends up being really costly to get even some basic data or analysis about what’s going on in the real world.
Do you ever compare computer vision to human eyesight?
Yes, because a great way to gauge how challenging a machine learning task will be is to measure how difficult it is for a human eye to make the decision. We create benchmarks for our models based on how accurate the current experts involved in the process are. Benchmarks for our particular tasks are typically only 85–90%, even for an expert who’s very familiar with the problem. So what that tells us is, ‘it is a hard problem because it is subjective’.

What is your opinion on the future potential of computer vision?
I think images are the most underutilised and biggest potential source of data that companies have. Tons of companies are using images in core parts of their operations, but they sit in storage pockets, so no one knows where they are or how to even use them. Using computer vision to unlock additional insights or efficiencies in a business is going to be massive. And I think the challenge is that image capturing, image processing, even video processing are all still quite technically challenging for a lot of teams.
What do you think is stopping companies from adopting these tools?
Well, I think we are just at the beginning and it’s hard to know what we’re technically capable of. The great thing about computer vision is that it’s very visual, so if you can show someone who is uploading an image how AI can make a decision, then suddenly they get it. But in order to do that as AI providers, we have big hurdles to jump over. Just getting access to the data needed to build really well-performing models is hard. Here we’re talking about really transforming a way of doing something, not just a little incremental improvement, but a really massive, massive change to their operation. And so, of course, that can be scary, but it can also be something that’s incredibly exciting.
Do you have an ideal, ‘dream’ solution for the micro-mobility use case, and if so what would it be?
The ideal solution will be the companies and cities working together with access to real-time data on their vehicles and cities. They should be able to proactively respond to the rapidly changing environment — for example, cities opening up new lanes for micro-mobility vehicles or companies restricting parking within certain areas that are overcrowded. In order to do this, you need to combine user-generated, vehicle IoT, and city infrastructure data together into one intelligent system.
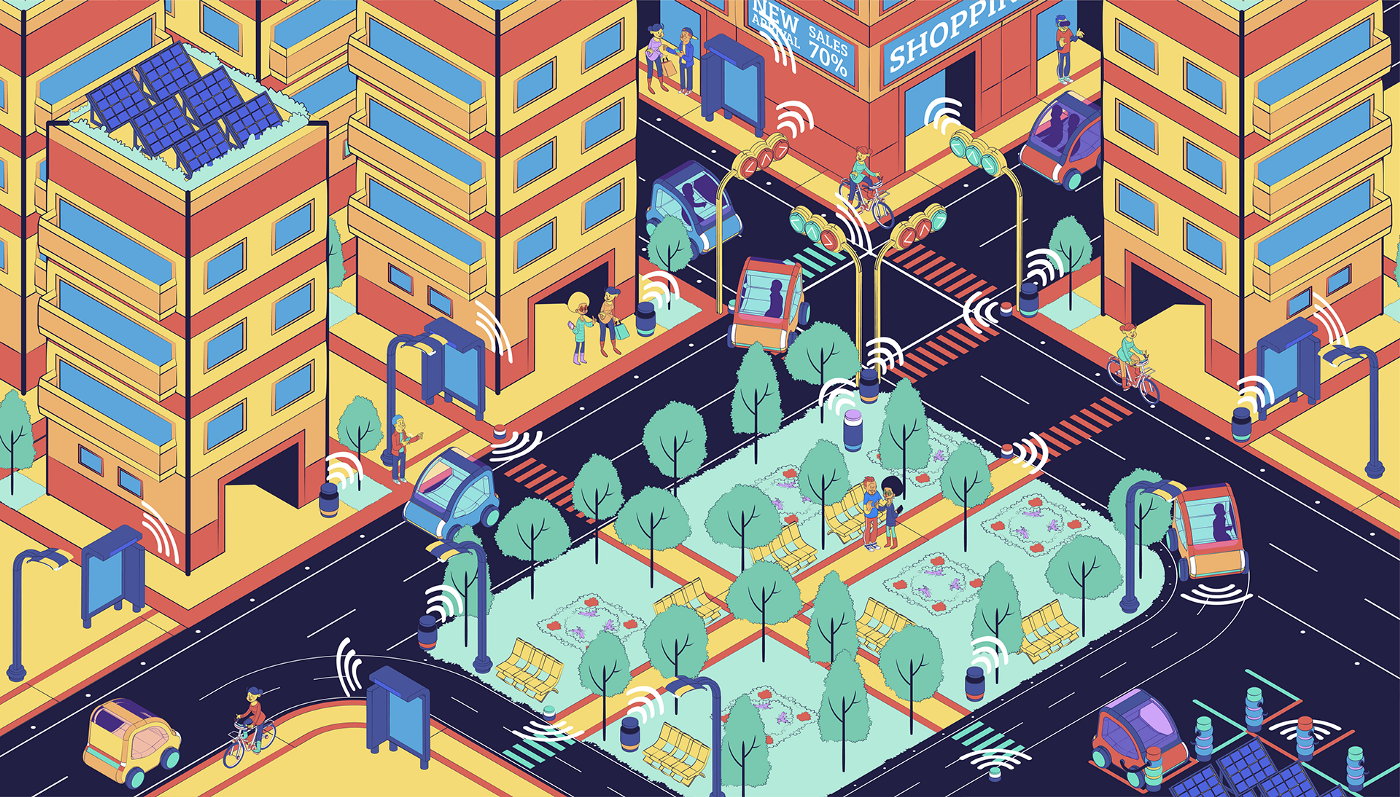
For more posts like these follow us on Twitter, LinkedIn and Medium. Stay tuned for more interviews and discussions with people working across the Trust & Safety and Brand Safety space.
At Unitary we build technology that enables safe and positive online experiences. Our goal is to understand the visual internet and create a transparent digital space.
For more information on what we do you can check out our website or email us at contact@unitary.ai.